香取勇一准教授がニューラルネットワークの国際会議IJCNN2019で最優秀論文賞を受賞
2019年7月14~19日にハンガリーのブダペストで開催された国際会議 International Joint Conference on Neural Networks (IJCNN2019)において、香取勇一准教授らの論文”Reservoir Computing Based on Dynamics of Pseudo-Billiard System in Hypercube”(超立方体上の疑似ビリヤード系ダイナミクスに基づくリザバー計算)が” Best Paper Award”(最優秀論文賞)を受賞しました。採択された800以上の論文から最も優れた論文として選ばれたものです。
IJCNNは、IEEEと国際ニューラルネットワーク協会(INNS)が共同で開催するニューラルネットワーク分野の世界有数の国際会議です。今年は世界80カ国以上からニューラルネットワークの研究者が集結し、ニューラルネットワークの理論と応用、計算論的神経科学、ロボティックス、分散知能等幅広い分野について活発にハイレベルな議論が繰り広げられました。
受賞対象となったのは、再帰型ニューラルネットワークの1つで時系列予測・パターン認識・ロボット制御など、様々な用途に応用可能なリザバー計算を、超立方体の内部で非線形素子が起こす複雑な反射運動(疑似ビリヤード系ダイナミクス)を用いて実現するという新しいアイデアです。これはデジタルとアナログの両方の良い特性を組み合わせたハイブリッド情報処理機構であり、効率的に電子回路などのハードウェアに実装することが可能です。本研究の成果は、非線形ダイナミクスの応用という観点から注目されるとともに、脳型人工知能の基盤技術となることが期待されています。
なお、本研究は、国立開発法人新エネルギー・産業技術総合開発機構(NEDO)の委託業務の結果得られたものです。
Reservoir Computing Based on Dynamics of Pseudo-Billiard System in Hypercube
Yuichi Katori 1, Hakaru Tamukoh 2, Takashi Morie 2
1: Future University Hakodate, 2: Kyushu Institute of Technology
Abstract—Reservoir computing (RC) is a framework for constructing recurrent neural networks with simple training rule and sparsely and randomly connected nonlinear units. The network (called reservoir) generates complex motion that can be used for many tasks including time series generation and prediction. We construct a reservoir based on the dynamics of the pseudo-billiard system that produce complex motion in a high-dimensional hypercube. In particular, we use the chaotic Boltzmann machine (CBM) whose units exhibit chaotic behavior in the hypercube. The units interact with each other in a time-domain manner through its binary state, and thus an efficient hardware implementation of the system is expected. In order to utilize the CBM as the reservoir, it is necessary to control its chaotic behavior for ensuring the echo state property of RC and establish encoding and decoding for input and output signal. For this purpose, we introduce a reference clock and analyze effects and properties of the reference input. We evaluate the proposed model on the time series generation tasks and show that the model works properly on a broad range of parameter values. Our approach presents a novel mechanism for time-domain information processing and a fundamental technology for a brain like artificial intelligence system.
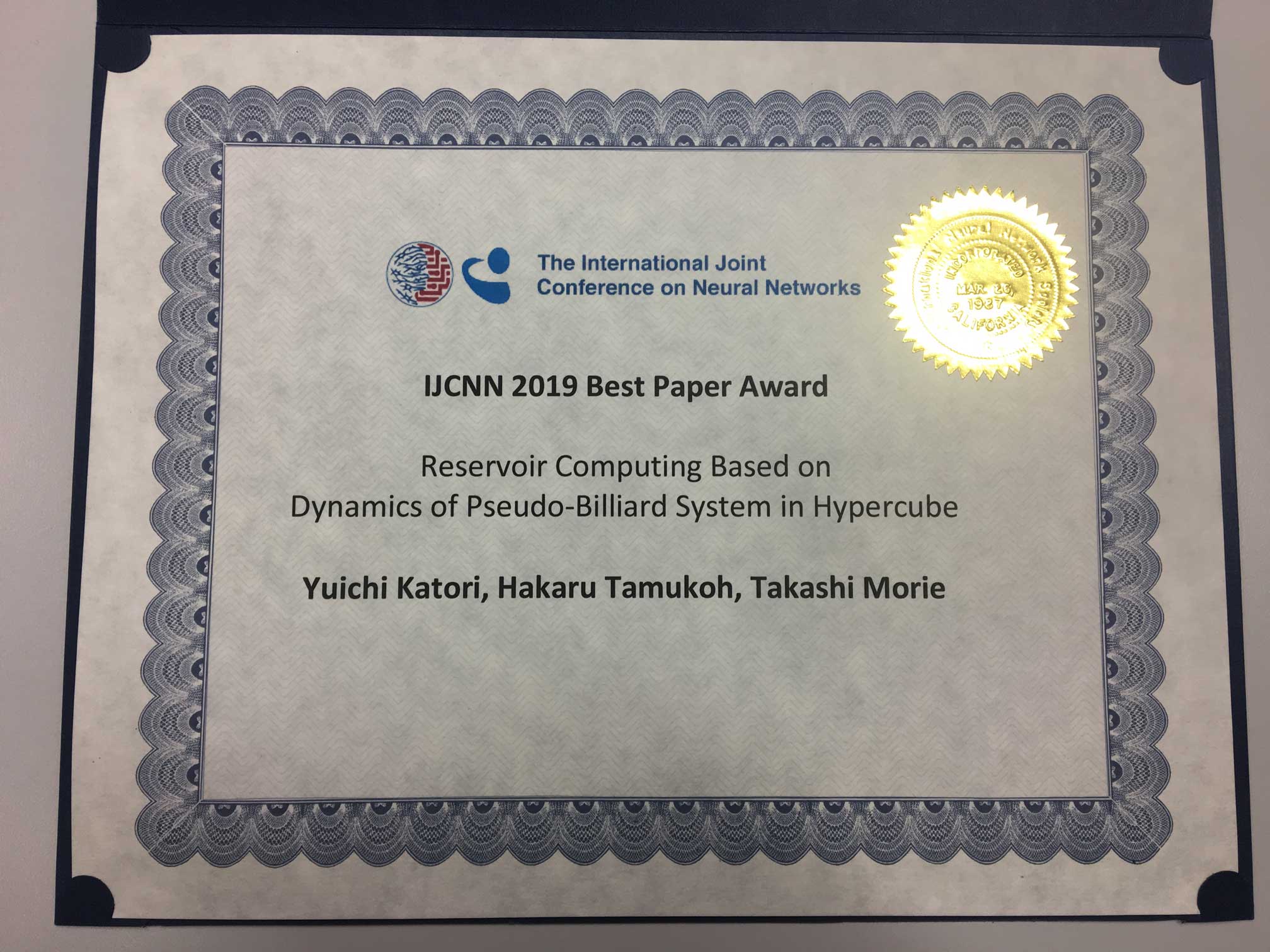