米村祥裕君(博士前期課程1年 香取研究室)が国際会議NOLTA2020で”Best Student Paper Award”(最優秀学生論文賞)を受賞
2020年11月に開催された国際会議International Symposium on Nonlinear Theory and Its Applications(NOLTA2020)において、米村祥裕(複雑系情報科学領域、指導教員:香取勇一准教授)が、マルチモーダル情報処理の階層的ネットワークモデルに関する下記の発表で”Best Student Paper Award”(最優秀学生論文賞)を受賞しました。採択された76の論文のなかから最も優れた論文として選ばれたものです。
“Multi-Modal Processing of Visual and Auditory Signals on Network Model Based on Predictive Coding and Reservoir Computing”(レザバー計算と予測符号化に基づくネットワークによる視覚・聴覚信号のマルチモーダル情報処理)
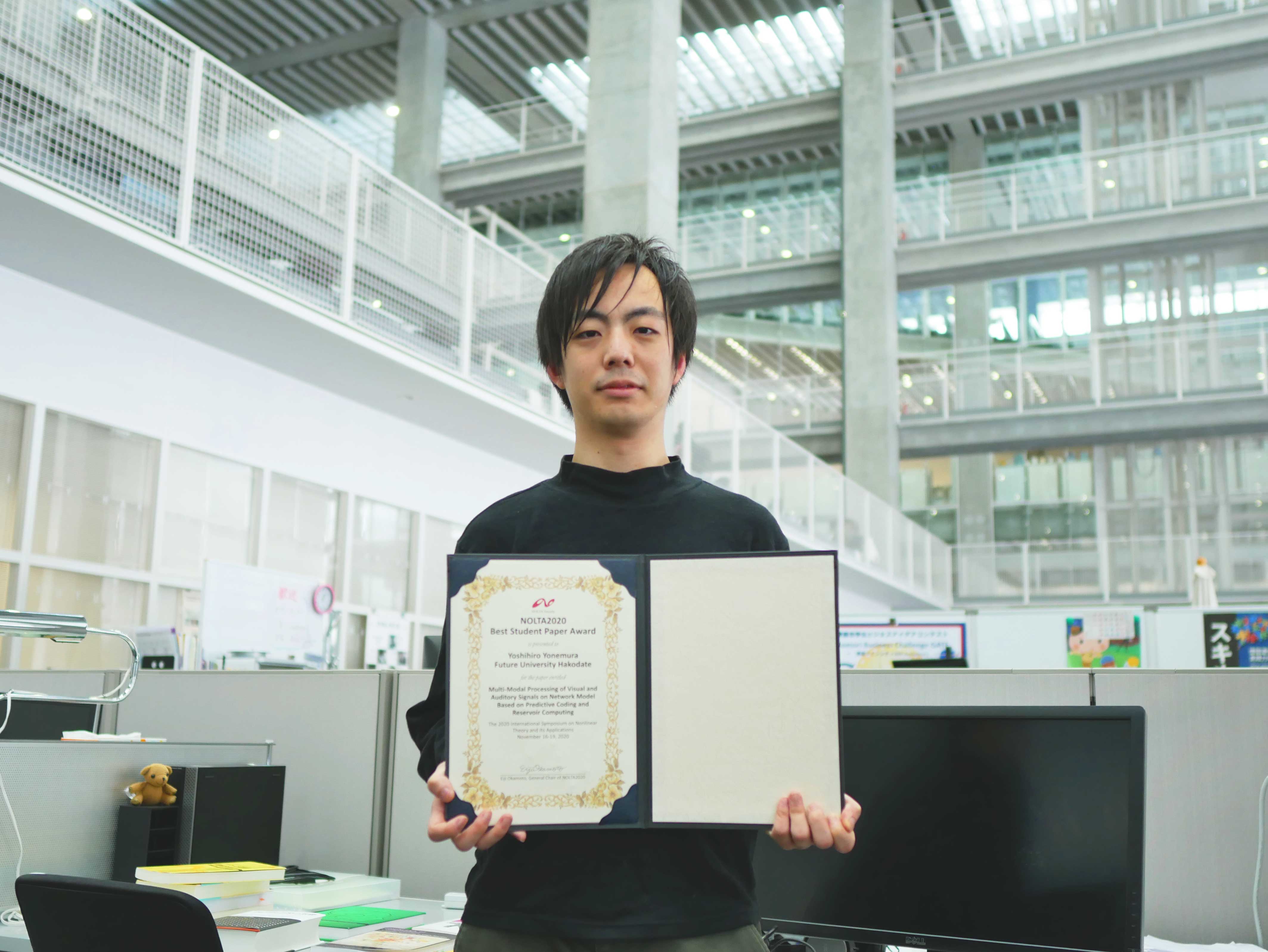
受賞対象となったのは、視覚情報や聴覚情報といった種別の異なる情報処理を行うマルチモーダル情報処理を、レザバー計算によって実装された動的な予測符号化モデルによって実現するという新しいアイデアです。マルチモーダル情報処理は人間の重要な能力の一つです。また、予測符号化は、脳の階層的な処理構造を説明する数理モデルとして様々な関心が持たれています。本研究の成果は、脳の情報処理モデルとしての観点から注目されるとともに、脳型人工知能の基盤技術としての発展が期待されています。
“Multi-Modal Processing of Visual and Auditory Signals on Network Model Based on Predictive Coding and Reservoir Computing”
Yoshihiro Yonemura 1, Yuichi Katori 1,2
1: Future University Hakodate, 2: Institute of Industrial Science The University of Tokyo
Abstract - We propose a hierarchical network model based on predictive coding and reservoir computing that integrates multi-modal sensory information. The network is composed of visual, auditory, and integration areas. In each area, the dynamical reservoir acts as a generative model that reproduces the time-varying sensory signal. The states of the visual and auditory reservoir are spatially compressed and are sent to the integration area. The model is trained with a dataset of time courses, including a pair of visual (hand-written characters) and auditory (read utterances) signal. We confirmed that the model reconstructs the visual signal from a given corresponding auditory signal. Our approach presents a novel dynamical mechanism of the multi-modal information processing in the brain and fundamental technology for a brain like artificial intelligence system.
この成果は、国立研究開発法人新エネルギー・産業技術総合開発機構(NEDO)の委託業務(JPNP16007)、およびJSPS科研費(20H04258)、JST,CREST(JPMJCR18K2)の結果得られたものです。
関連リンク: